
Industry 4.0 or Lean 4.0?
INTERVIEW – Atlantis Foundries was able to achieve zero defects for three months in a row thanks to machine learning. Here’s why the human component can’t be discounted.
Interviewee: Pieter du Plessis, former CEO, Atlantis Foundries – Cape Town.
Interviewer: Anton Grütter, Director, Lean Institute Africa – Cape Town.
Cape Town is sometimes called the “Silicon Valley of South Africa”. Yet, it was a pleasant surprise to learn that Atlantis Foundries became not only an African, but a global leader in the foundry industry using AI and machine learning to achieve zero defects on the truck engine block castings that they delivered to their customers in Europe and the United States.
In this interview Pieter du Plessis, the CEO of Atlantis Foundries at the time of this initiative, tells the story of how they used AI to solve a strategic business problem that you can read or view below. Given the hype about Industry 4.0, there are some valuable lessons in here from someone who actually made AI work in the real world.
There is much to learn from what Pieter shares with us, but I would like to highlight one aspect in particular. It has become fashionable to talk about going “beyond lean” in the rush to market Industry 4.0 technologies. To be sure, these new technologies are game-changing and cannot be ignored, but I think that referring to them as “beyond lean” is the result of a fundamental misunderstanding of what lean thinking is about.
Before introducing AI, Atlantis Foundries invested in continuous improvement for many years to improve their casting defect rate from very poor to industry standard. This meant that, by the time they started experimenting with AI, there was already a problem-solving culture in the organization that they could build on. You will hear Pieter refer to AI as another “tool” in the improvement journey. In other words, just like we use 5 Whys as a tool to find root causes, there is now this very powerful tool called AI that can be used in situations where humans cannot cope with the amount of information that needs to be processed.
Like any other problem-solving tool, AI can be used incorrectly or inappropriately if you do not apply lean to the problem at hand. Asking what issue we need to solve has to be the first step, which will inform our problem-solving and make it effective. Atlantis Foundries thought very carefully about this question and that guided them as they figure out how to tap into AI.
This story shows us how important lean thinking is in making a success of new technologies like machine learning and AI.
Anton Grütter: Pieter, can you introduce us to Atlantis Foundries?
Pieter du Plessis: Atlantis Foundries is a spin-off of Atlantis Diesel Engines, an engine plant bought in the late 1970s. The brand then died away, but the foundry was bought by Daimler/Mercedes Benz to continue to cast cylinder blocks for trucks. Daimler has a foundry in Mannheim in Germany, and with the severe environmental laws in Germany they couldn’t expand their capacity. They were bought by Daimler in 1999 to make these castings. Atlantis Foundries exported all its engines to customer in the UK, USA, South Kores and Germany.
AG: Can you please explain to us why quality was so important to Atlantis Foundries?
PDP: Being so far away from the customer we served meant that, if you have any quality problems detected at the machining operations in the customer’s plant, the whole pipeline could potentially be contaminated. The cost of looking for quality defects in the pipeline – which could take six to eight weeks – is enormous. That’s why first-time right quality was so critical for us. Our quality costs were significant.
AG: How did you try to address this quality problem initially?
PDP: With the classical quality tools, like statistical process control, control plans, process sheets, and so on. Anything that traditional companies would do to improve quality. Yet, we reached a plateau, because the feedback loop was so long and the process was so complicated and full of variables. To find a pattern in those variables was difficult. A benchmark foundry making the parts that we made was typically running on 4-8% scrap, and we were running at around 6% - with an extra 1.5% at the customer’s site. We were mid-range.
AG: What made you decide to give machine learning a try?
PDP: We wanted to predict which component would be defective after machining in the US or Germany, and not ship it in the first place. Because your defect is a function of your process, there must be a pattern in your process that can be recognized. This way you know that, if the process is in a certain way, it will produce a defective casting. We are talking about defects that are below the surface of the cast and can’t be seen physically, nor found with ultrasonic methods because of the sheer size and volume. We wanted to predict, based on what the process was at that point in time, whether a casting would be scrap or not. At the end, we had a 70% success rate. We would say, throw these 10 castings away and we knew that seven would be scrap. We knew we’d throw three good ones away, but the cost associated was insignificant compared to what casting in the US.
AG: How long did you make that decision?
PDP: We started with machine learning in March 2017, giving Data Prophet [machine learning specialists in Cape Town] 18 months’ worth of data of the process and of the quality results (both internally and externally).
We didn’t have to generate new data. We only took the data that we had on a daily basis, which we never used. The most difficult task is matching the data to the component you make: we normally use time series – checking the process every half an hour, 40 minutes or once a day – but we still made a component every minute. We used to make 700 components a day, an enormous number if you think about it. Out of 180,000 truck engines built, at first we only had perfectly matching data for 7,000 blocks. So, we used that data to build the first model. As you feed in more data every week, the algorithm learns again. You can also start to do your testing to match exact components, which is quite critical for the process: when we make block number 1 or number 20, we know what parameters we should be checking, as opposed to randomly check at regular intervals. Essentially, our testing methods changed.
AG: How does this work in practice?
PDP: There are various types of artificial intelligence and machine learning. There are supervised and unsupervised learning. With unsupervised learning, the answer is not known; you give the machine the raw data and it finds the patterns in there. With supervised learning, we tell the machine whether the outcome was a good or a bad block and provide the process parameters for each; the algorithm uses brute force by calculating all iterations until it gets to an answer (however, we had 185 process variables, so you can imagine the complexity).
AG: Were there problems with people? Did they accept this?
PDP: We did have problems. There were many non-believers, including me. I was very skeptical at the beginning, because I didn’t understand how the specialists could just request data without understanding our process. Some of the engineers feared for their job, and thought AI was going to replace them. I underestimated that, as I thought clever, well-educated people wouldn’t be scared of AI. It took a while for people to understand it and use it, but the results were spectacular.
Using the model, we wanted to predict which block would be scrap in the US and, in the second phase, learn what we had to do to avoid making scrap in the first place. It went from predicting the scrap to prescribing the process. It took process parameters and calculated the region for them all (min 11.), which meant that our process limits were squeezed significantly and some of them were adjusted. The combination of these two things allowed us, in the second half of 2017, to achieve zero defects in three plants for three months in a row. This is unheard of.
I have spoken to some of the guys recently, and that trend is continuing – even though zero defects is not achievable all the time, because process parameters become so tight over time. And that’s why people will always be important in this AI thing: they will have to look at this report and decide what they need to do to the machine to enable it to achieve the desired outcome. You can’t tell a machine, “You can’t do that.” Machines are not that clever. The notion that AI will replace all humans is a fallacy. Maybe in a couple of centuries. Certainly much later than many people think.
AG: What advice do you have for people interested in Industry 4.0?
PDP: I think a lot of people are struggling with what Industry 4.0 really means. They say, “We have data, let’s do something with it.” For me, that approach is wrong. They should instead ask themselves what the biggest problem in their business is and how this additional tool can help them to achieve their goal. At Atlantis Foundries, for example, we wanted to prevent a scrap black in the US or Germany. Once we were able to predict which block would be defective, we wanted to figure out what we had to do to prevent it in the first place. You need a clear goal. Just doing Industry 4.0 for the sake of it is a waste of time.
AG: More and more are talking about going “beyond lean” these days when they discuss technologies like AI. What do you think about that?
PDP: In my mind, lean is about streamlining processes, reducing waste and making the work repeatable and efficient. To me, AI is another tool enabling you to do that. It’s not a silver bullet; it’s just another tool. As I got more involved in it, I quickly realized how far we are from machines doing everything by themselves with no people involvement necessary. We are incredibly far from that. The human aspect is important, because part of the process is human influences. If an operator doesn’t follow the rules, you can have all the AI in the world but you are still going to have a problem. So, for me, AI is not beyond lean… it’s another tool for lean.
THE INTERVIEWEE

THE INTERVIEWER

Read more
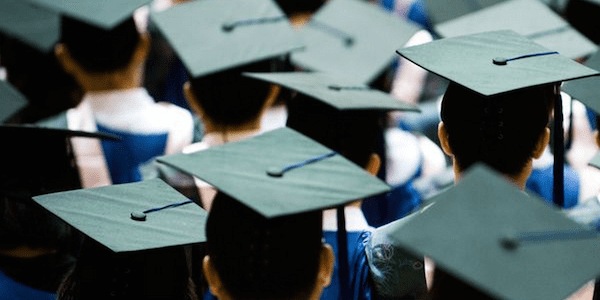

INTERVIEW - Planet Lean speaks with Constantin May, Academic Director of the CEPTM institute at Germany’s Ansbach University, which organized the first Lean Educator Conference in Europe in co-operation with LGN.
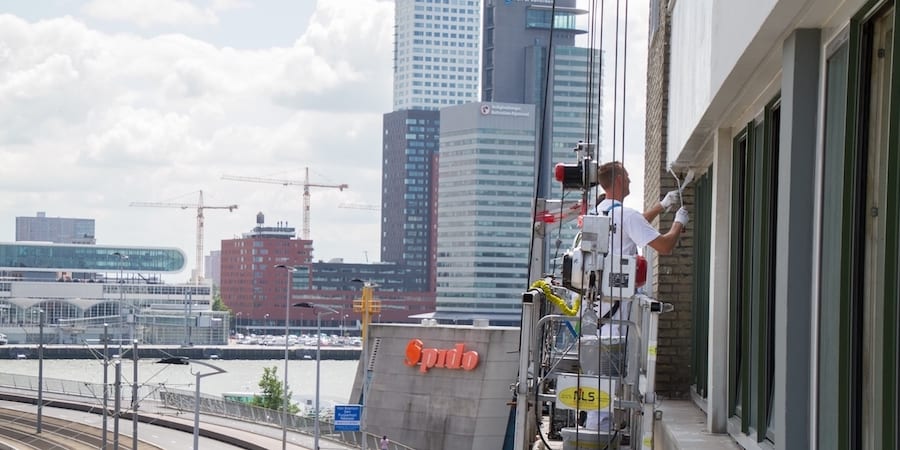

FEATURE – Visualizing the work, increasing customer focus and changing the way to interact with employees has helped a family-run Dutch building maintenance and renovation firm to transform itself.



OPINION – The digital revolution has forever changed the nature of employment: today's workers have more choice and employers competing for them than ever before. It's about time our approach to HR reflected that.


FEATURE – If you think one is born a leader, think again. Leadership can be learned and, in this article, the author provides a four-step guide to developing it.